Supply chains today face unprecedented risks that can severely disrupt business operations. From geopolitical instability to climate change, the potential threats are complex and dynamic. This creates massive challenges for organizations in identifying and mitigating supplier-related risks. Traditional supplier risk assessment methods relying heavily on surveys, audits and human analysis are inadequate. They often fail to detect emerging risks in time.
At the same time, artificial intelligence adoption in supply chain operations has accelerated, with 62% of companies already using AI for sustainability tracking and measurement according to an EY study. However, generative AI opens up even more disruptive potential that is just beginning to be explored. This is where generative AI, specifically, promises to be transformative for supplier risk assessment. By synthesizing and analyzing massive amounts of structured and unstructured data, generative AI models can continuously monitor suppliers and provide real-time risk alerts. As the technology matures, generative AI’s ability to simulate hypothetical scenarios will strengthen predictive capabilities beyond what is possible today.
Organizations that leverage generative AI for supplier risk assessment will gain significant competitive advantage through more resilient supply chains and informed risk mitigation strategies. Already, 40% of supply chain organizations are investing in generative AI, focusing on knowledge management applications according to EY research.
The AI revolution is here. Leaders who embrace generative AI for supplier risk assessment will future-proof their supply chains against disruption.
The Growing Criticality of Supplier Risk Assessment
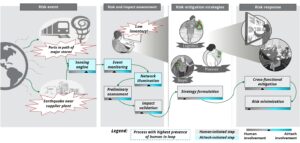
With globalized and just-in-time supply chains, businesses are highly vulnerable to disruptions. A single supplier failure can halt entire production lines, leading to substantial revenue losses. Supplier risks arise from various factors, including financial problems, labor issues, compliance breaches, natural disasters, geopolitical instability and more. Climate change is introducing new risks of rising frequency and severity.
Unfortunately, traditional supplier risk assessment methods are inadequate in today’s volatile environment. Surveys and audits only provide periodic snapshots that miss fast-emerging risks. Data silos make it hard to connect insights across suppliers. The volume and complexity of risk factors overwhelm manual analysis. The need for more sophisticated, continuous and data-driven approaches is urgent.
How Generative AI is Transforming Risk Assessment
Traditional supplier risk assessment relies on periodic surveys, audits, and manual analysis by human teams. This approach has key limitations:
- Assessments only provide snapshot vs continuous view of risks
- Lagging indicators fail to detect emerging risks in time
- Human capacity constrains data processing volume
- Silos limit cross-validation of risk factors
- Hypothetical scenarios cannot be modeled for predictive insights
Generative AI overcomes these hurdles through machine learning techniques. It synthesizes vast, disparate data streams in real-time to identify subtle risk indicators across thousands of suppliers. By analyzing leading indicators, generative AI provides early warning on emerging issues.
Advanced models can also simulate hypothetical scenarios to stress test supplier networks against disruptions. This reveals vulnerabilities and quantifies resilience. Generative AI additionally generates tailored, optimized risk mitigation recommendations accounting for business constraints.
Where human-led methods are periodic, siloed, reactive and limited in analytics capabilities – generative AI enables continuous, holistic, predictive and nuanced risk assessment. It transforms supplier risk management from fragmented and backwards-looking to integrated and forward-looking.
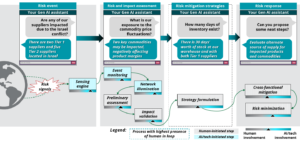
The Benefits of Integrating Generative AI
Traditional supplier risk assessment relies primarily on periodic surveys, audits, and manual analysis by supply chain analysts. This approach has significant limitations including: narrow datasets confined to supplier-provided data that can conceal risks; siloed analysis of individual suppliers that inhibits cross-validation to detect correlations; static assessments providing only periodic snapshots and missing emerging risks in between; reliance on lagging indicators versus leading predictors of disruptions; bottlenecks in human analytical capacity restricting sophistication; standardized mitigation recommendations rather than tailored strategies; and capped scalability based on limited analyst bandwidth.
Fundamentally, traditional methods are fragmented, reactive, and constrained in leveraging data and analytics to deliver dynamic, tailored, predictive risk management. The constraints of manual analysis create barriers to catching and responding to risks in an agile manner.
Generative AI overcomes the limitations of traditional methods through:
Holistic datasets: AI synthesizes structured supplier data with unstructured news, social media, regulatory filings and other external sources for 360-degree visibility.
Correlation detection: Machine learning uncovers risk interconnections across thousands of suppliers that evade human analysis.
Continuous monitoring: AI continuously ingests streams of supplier data to provide 24/7 visibility rather than periodic snapshots.
Leading indicators: Natural language processing analyzes alternative sources to detect early signals of potential disruptions.
Predictive modeling: AI can run simulations of hypothetical scenarios to estimate impacts and stress test contingency plans.
Custom recommendations: Generated risk management plans account for business priorities, risk tolerance, resources and constraints.
Scalability: AI assessment quality remains consistent irrespective of supplier base size.
Generative AI in Action
- Unilever is a prime example that uses generative AI to optimize manufacturing, distribution, and logistics, resulting in significant cost savings and improved sustainability by identifying inefficiencies across the value chain. Read here
- Global shipping giant Maersk employs generative AI for strategic risk management by continuously monitoring shipping routes, weather patterns, geopolitical events, and simulating disruption scenarios to proactively adjust routes and minimize impacts. Read here
- UPS leverages generative AI to dynamically optimize delivery truck routing and load planning based on real-time traffic, weather, road conditions, package weights, and customer requirements – minimizing fuel use and transit times. Read here
Modeling hypothetical scenarios allows companies to stress test supply networks against disruptions like natural disasters, demand fluctuations, and develop contingency plans – building resilience.
At Unilever and Maersk, generative AI enabled data-driven decisions through comprehensive analysis beyond human capabilities by synthesizing insights across disparate data sources.
Overcoming Implementation Challenges
Data Quality: Garbage in, garbage out. Generative AI models require massive amounts of high-quality, relevant data for effective training. Incomplete, outdated or erroneous data will lead to inaccurate outputs.
Explainability: Many AI models are black boxes – their reasoning is opaque. Explainable AI solutions that provide transparency into how recommendations are generated build trust.
Process Alignment: AI-driven risk alerts and recommendations are ineffective if disconnected from actual decision-making processes. Integrating AI insights into existing workflows is key.
Building Trust: Executives and stakeholders may be hesitant to rely on machine-generated advice for critical risk decisions. Proven performance builds confidence over time.
Start-Up Effort: Deploying generative AI is not plug-and-play. Upfront work like data preparation, model training, and integration requires dedicated resources.
Cost: While AI costs are dropping, purchasing solutions, computational resources, personnel and change management still involve significant investment.
The Future of Risk Management
As generative AI grows more powerful, its applications in supplier risk assessment will continue expanding. With enough quality data, AI models can one day simulate detailed disruption scenarios to estimate costs, develop contingency plans and build strategic resilience.
By complementing human intelligence with AI, supply chain professionals can devote more strategic efforts to strengthening relationships, ethics and sustainability versus reactive risk response. Shared visibility into risks further allows suppliers and buyers to collaborate on effective mitigation.
Generative AI remains in a nascent stage today. But maturing capabilities will make end-to-end supply chain transparency, agility and resilience a reality. Organizations that wait to embrace AI may find themselves losing competitive edge to forward-thinking peers.
Conclusion
Supplier risk assessment is undergoing a generative AI revolution. By enabling real-time monitoring, simulations and holistic analysis, AI empowers more proactive, data-driven risk management. As generative models grow more sophisticated, organizations can achieve breakthroughs in supply chain resilience and continuity. Though adoption faces hurdles, the capabilities unlocked are substantial and confer competitive advantage. Now is the time for supply chain leaders to take the plunge into generative AI for future-proofing risk management strategies.